Introducing Mr. Poopy Face
What is the average poop? What drives the differences between poop? Can we reconstruct poop? In facial recognition, a previous common method to gain a sense of someone's face is by understanding the average human face - and how that varies form person to the next. This method is commonly known as eigenfaces where it is possible to break down specific features of a face and how they change across people in general.
Before we can create Mr. Poopy Face - we need to first introduce the concept of principal component analyses. It is a very common method for dimensionality reduction which is helpful... cause we can only see three dimensions. PCA is a great tool to use for image analysis to understand your data. PCA is basaically done by assessing the variance of the data set by looking at the direction of spread (eigenvectors) and how much they spread by (eigenvalues).
It is generally a good idea to understand the mathematics behind these operations - especially since it is relatively simple and a good primer for what is ahead. Here are the steps:
1. Normalize based on normal distribution: (x - mean)/SD
2. Calculate the Covariance Matrix
3. Find the eigenvectors
4. Find the eigenvalues
5. Rank eigenvectors by eigenvalues
6. Project original dataset into the new space of the principal components
7. Plot them
A little explanation.
You standardize the data such that you center everything. This is a good framework to understand how the data moves. You then want to standardize each feature so that all of the variables are on the same paying field. This makes sense when you think of what you are doing when you are trying to find the eigenvectors of a covariance matrix. You are evaluating the amount of variance of how the data moves. And the highest eigenvalues will be associated with the highest variance of the dataset. Now - let's say I have the same exact dataset as my buddy except that I measured something by inches while my buddy measured something with centimeters. We have the same data except his data is scaled by 2.54. So when we look at the variance of that data, his will look to be more signifigant than mine - misleading what is really causing the highest spread of the data.
Now we look into calculating the covariance matrix but first what is it? Well it is the joint varability between two variables. Essentially let's say we have variable x1 and variable x2. When we increase variable x1 - we will ask ourselves - what happens to variable x2? Well if the covariance is greater than 0, then you would expect it to increase with x1. Basically it is correlation without dividing for standard deviation of the population of the data for both x1 and x2. To construct the complete matrix, we do this for the rest of the variables that we have. What will it end up looking like? A symmetric matrix. For simplicity sake, we will just pretend our image just has 3 columns of data as I want to explain how our three columns will produce 3 new eigenvectors that is going to be our new basis. And then we can calculate the covariance matrix just by a simple python command as well.
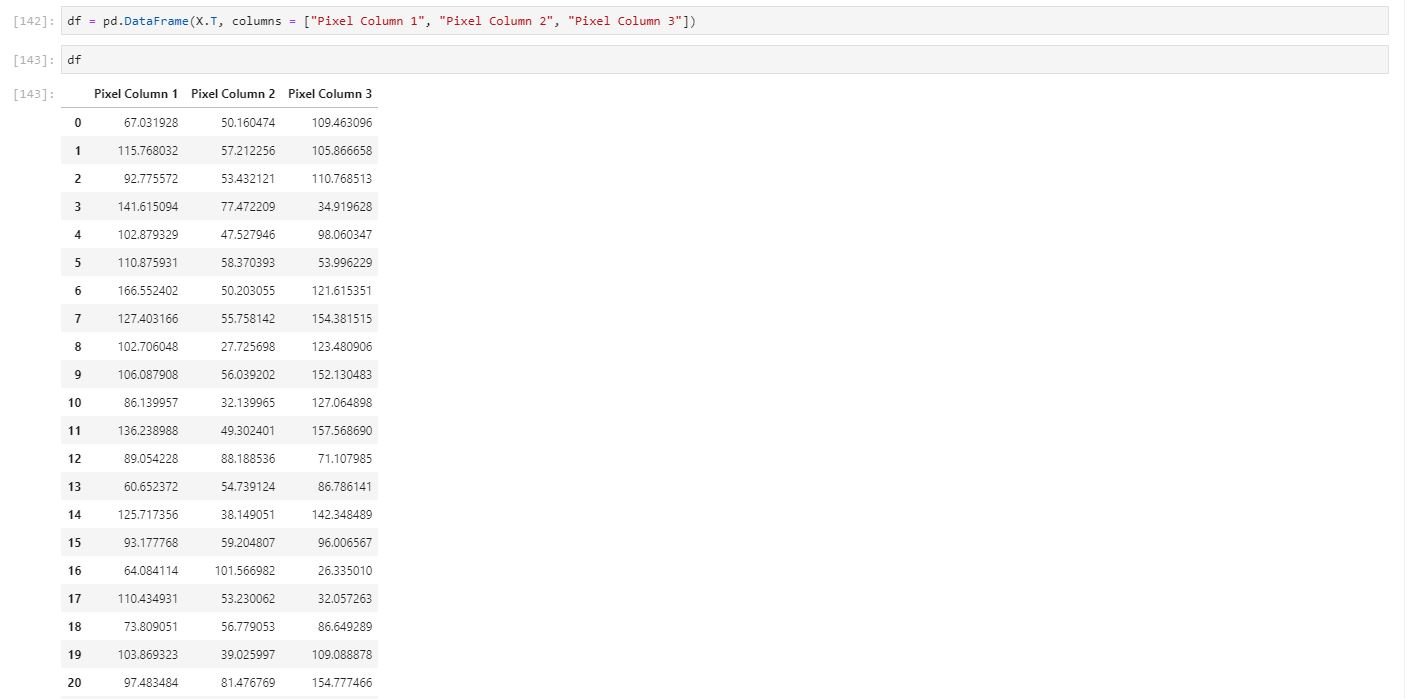
Covariance Matrix:

And now we can easily find the eigenvalues and eigenvectors. The number of eigenvectors will be how much variables there were present - if you have 1000 variables, you will have 1000 eigenvectors. In our case, we will only produce three. In most scenarios, calculating it by hand can be labor intensive and pretty unrealistic - especialy if it reaches to 1000 variables. But it is important to kind of understand what is happening here when you try to find the eigenvectors. What we are doing is performing a linear transformation from our original dataset space to another space that explains covariance. When this happens, some of the data will simply be a scalar transformation from our original dataset to the new space. Simply, when we transformed one of the data in our original space, the new transformed data was simply scaled on that specific vector by either 2, -7, and etc. That scalar value is our eigenvalues. And when we look to evaluate the eigenvalues and eigenvectors of the covariance matrix - we are looking at the magntiude of that scalar. Again, for python - it is a simple command and we can calculate our eigenvectors/values from our covariance matrix.
Now we basically have 3 eigenvectors and 3 eigenvalues. We can choose now to rank them from high to low, low to high, or some other way you want to describe the data. It doesn't have to be max eigenvalues! Although that is usually the most popular and usually more useful. So let's say we want to visualize our new data in two dimensions. Easy let's pick our two eigenvectors (based on max eigenvalues) and multiple it to our original dataset subtracted by average mean vector. Now we have transformed our dataset into the space of principal components. In reality, this is simply a change of basis from what we normally consider standard (i component with a unit vector in only the x direction, j component with a unit vector in only the y direction, and k component with a unit vector in only the z direction. I am using a function from a repository made by Barba Lab (https://github.com/engineersCode/EngComp4_landlinear) that quickly shows how after we find our eigenvectors, we can change the basis to the three eigenvectors we have so that we are now in the space of how our data moves in accordance to principal components and from these three - we can reduce the dimension to two (x and y) or one dimension if we so choose.
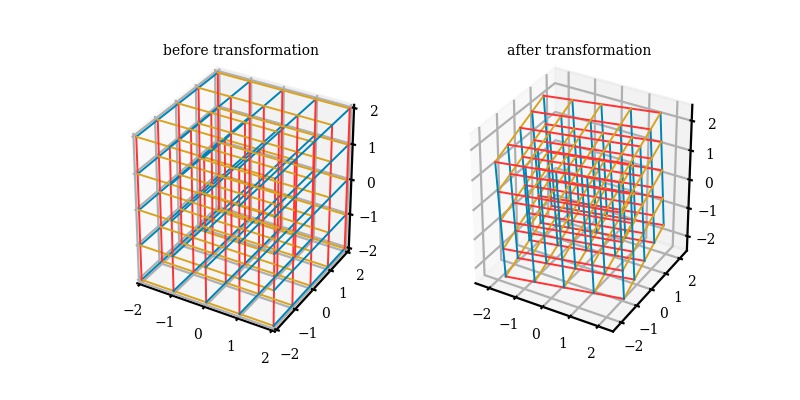
This is all pretty high-level stuff and I would recommend digging through a textbook. I recommend Introduction to Linear Algebra by Gilbert Strang or immersive linear algebra (http://immersivemath.com/ila/index.html) to get a better understanding and nuances you should consider but now to the good part. Once we understood how PCA decomposes our dataset into varability, it is easier to explain why it matters for poop. I kind of gave a primer that poop has different textures and etc but is usually represented by a classification such as the Bristol Stool Chart. What if we take 1000s of images of poop and evaluate the PCA of them? You would essentially get eigenvectors or "modes" where you have the highest variation in data. With this, we can look into classifying stool based on eigenvectors - and let me clarify again, you don't always need to classify based on max variation. Likely the first three with the highest magnitutde might not be a good method to actually classify.
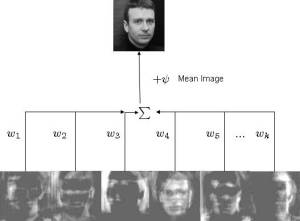
This principle idea is used in some facial recognition algorithims - commonly referred as eigenfaces. In quick summary, we aim to find the "poop" face of specific stool classifications. What is normally done is that you will have an image that is N X N but you will make that image into an N2 X 1 vector instead. Once you have this, you can now make a stack of images into a matrix of N2 X images. First though, we will resize all of our images to 250 X 250 matrix such that N2 will be equal to 62,500 such that this dataset is manageable. I will also only use 20 images as purely an example - and as such our matrix will be 62,500 X 20. Then we calculate a new vector that will be the mean of the 20 images from each pixel value. We subtract each of the 20 image vectors by the mean vector - such that we have centered the data. Once we have that, we can calculate the covariance matrix of this dataset. Usually this is computationally expensive though and single value decomposition (SVD) will be used instead (there are multiple ways to calculate the eigenvectors/values). I won't explain it much in detail but essentially it is another method to decompose our mean subtracted dataset.
Fortunately, SVD is pretty much a single line code operation with the numpy library and with this we can get our eigenpoops. Below is an image of the mean vector that we had calculated with the eigenvectors that is ordered by eigenvalues or variability based on the covariance matrix. I decided not to blur it this time as it is fairly undistingushable from the daily poo.
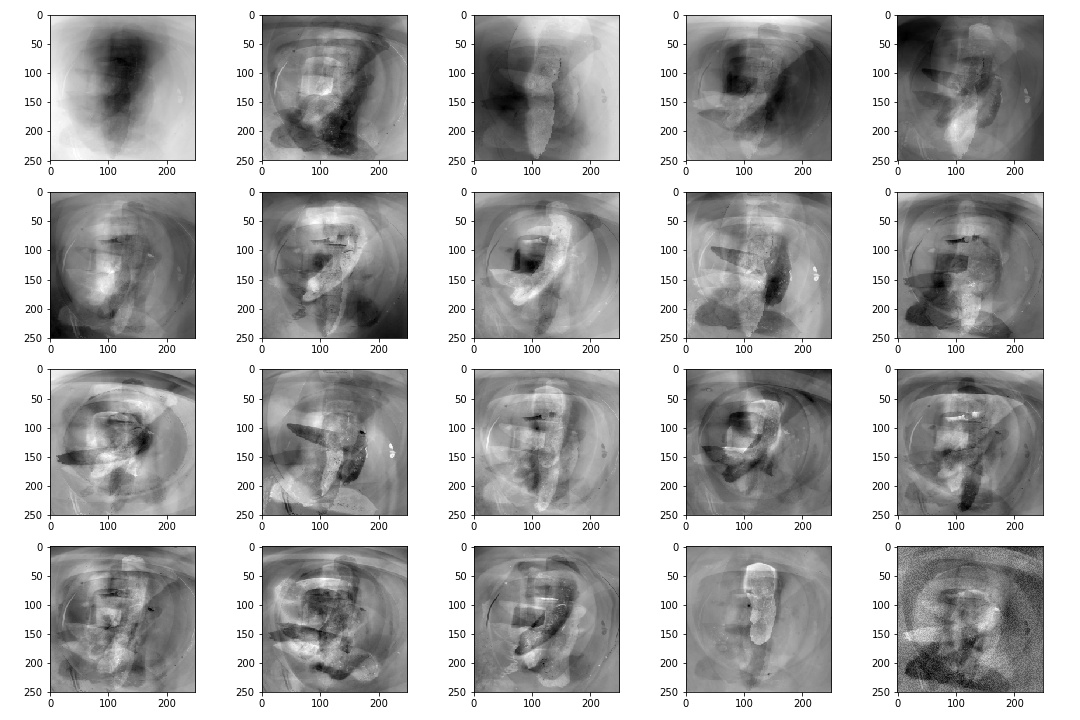
Yet, these eigenvectors can be very important and be used to classify different varaitions in the poop images. This could be used as tool to classify more finer details of let's say a bristol stool type 1 classification. Bristol stool is a good option but maybe it could be better? We plan to extend the image analysis capability of stool and this can be an important method to doing so. And I would like to clarify once again that maybe the plotting the eigenvectors associated with highest eigenvalues may not be a good option. Maybe it would be better to look at the 10th ranked eigenvector or the 17th. These eigenvectors may do a better job in classifying variation in bristiol stool type 1s. There are also other opportunities in using these 'eigenpoops' where we can re-construct new stool images. This may be a good to take advantage of in the future if I figure out a good way of applying it to the problem scope but for now, this aim is to find varability with stool classifications.